K-Means Classifier¶
The job of the K-Means Classifier is to establish \(k\) nodes, each one representing the “center” of a cluster of data. For each node desired then, the algorithm positions that center (called a “centroid”) at the point where the distance between it and the nearest points is on average smaller than the distance between those points and the next node.
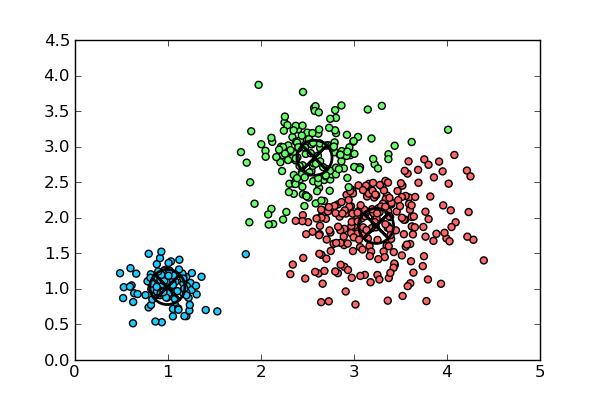
The Algorithm¶
- Within the space of your data, choose \(k\) nodes to have random positions.
- Calculate the distance between every data point and each node.
- For each data point, assign its “class” to whichever node is closest.
- Using these tentative classes, find the average position (i.e. the mean) of each class. Basically, where’s the “center” of each class?
- Move each node to the position of its class’s center.
- Repeat steps 2-5 until the nodes don’t move much anymore or until you’ve reached some maximum number of iterations.
- Assign the resulting classes to the data.
Advantages¶
- Will always result in a solution.
- Generally good at picking centroids when data shows believable separations.
Disadvantages¶
- Computationally expensive for large data sets
- Highly dependent on how data is clustered. Also highly dependent on what data is present. Under-sampling skews results.
- Highly dependent on where centroids are initialized. Problematic when they’re close to each other.
- Highly dependent on how many centroids are initialized.
- Number of centroids may not correspond to actual number of separations in data; entirely at the mercy of the programmer.
- Poor at predicting separations in irregularly shaped data (e.g. elongated clusters)
Attributes¶
- centroids: the number of clusters expected from the data
- max_iter: the number of iterations before the algorithm stops
- min_step: the smallest difference in distance between an old centroid and a new centroid before the algorithm stops
Operations¶
- clf.fit(full_data, k): generate \(k\) nodes for classifying data.
- clf.predict(some_data): predict classification (i.e. if \(k = 2\) then class 1 or 2) on data.